Hexxcell Webinars
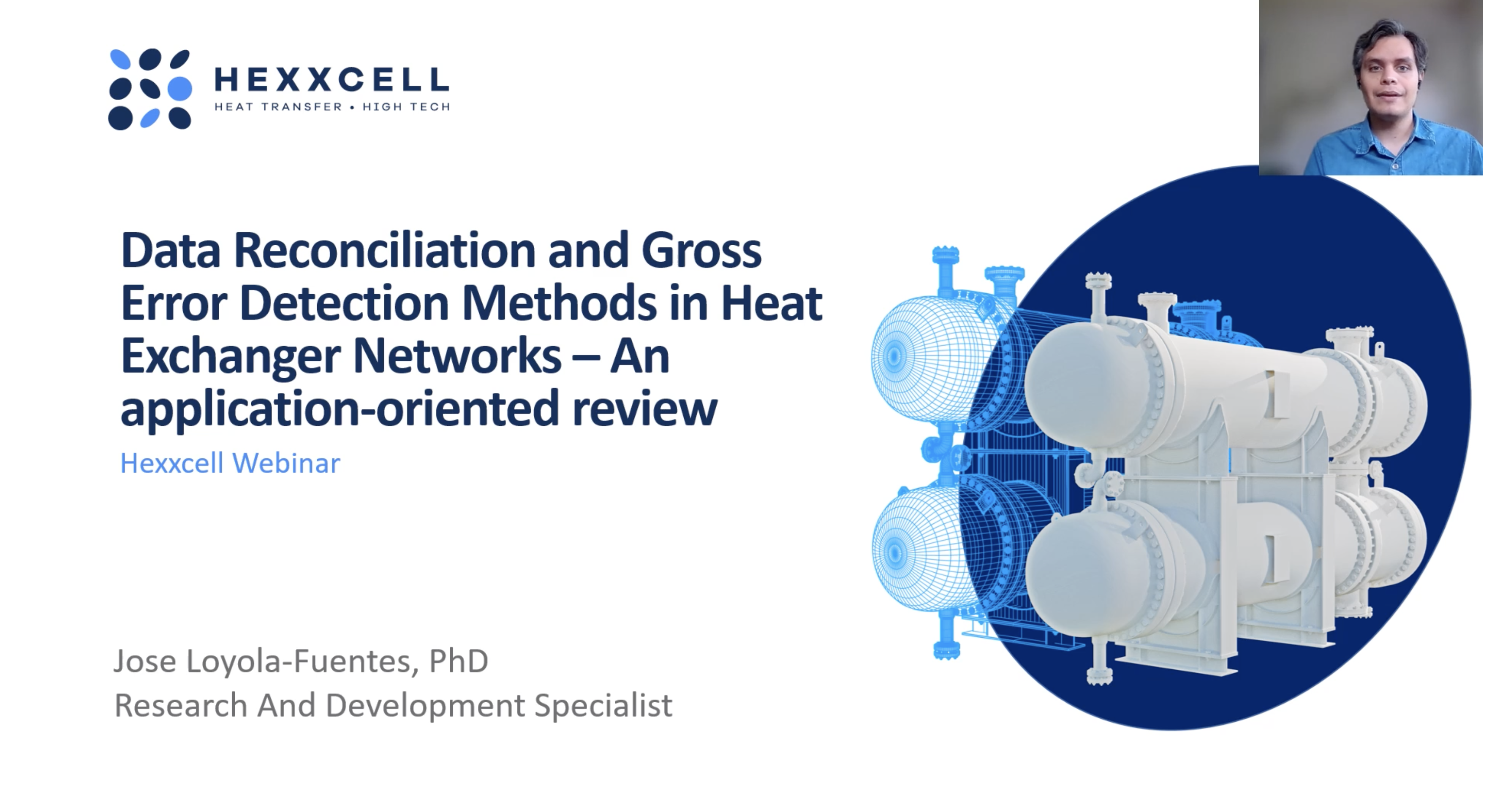
Data Reconciliation and Gross Error Detection Methods in Heat Exchanger Networks – an application-oriented review
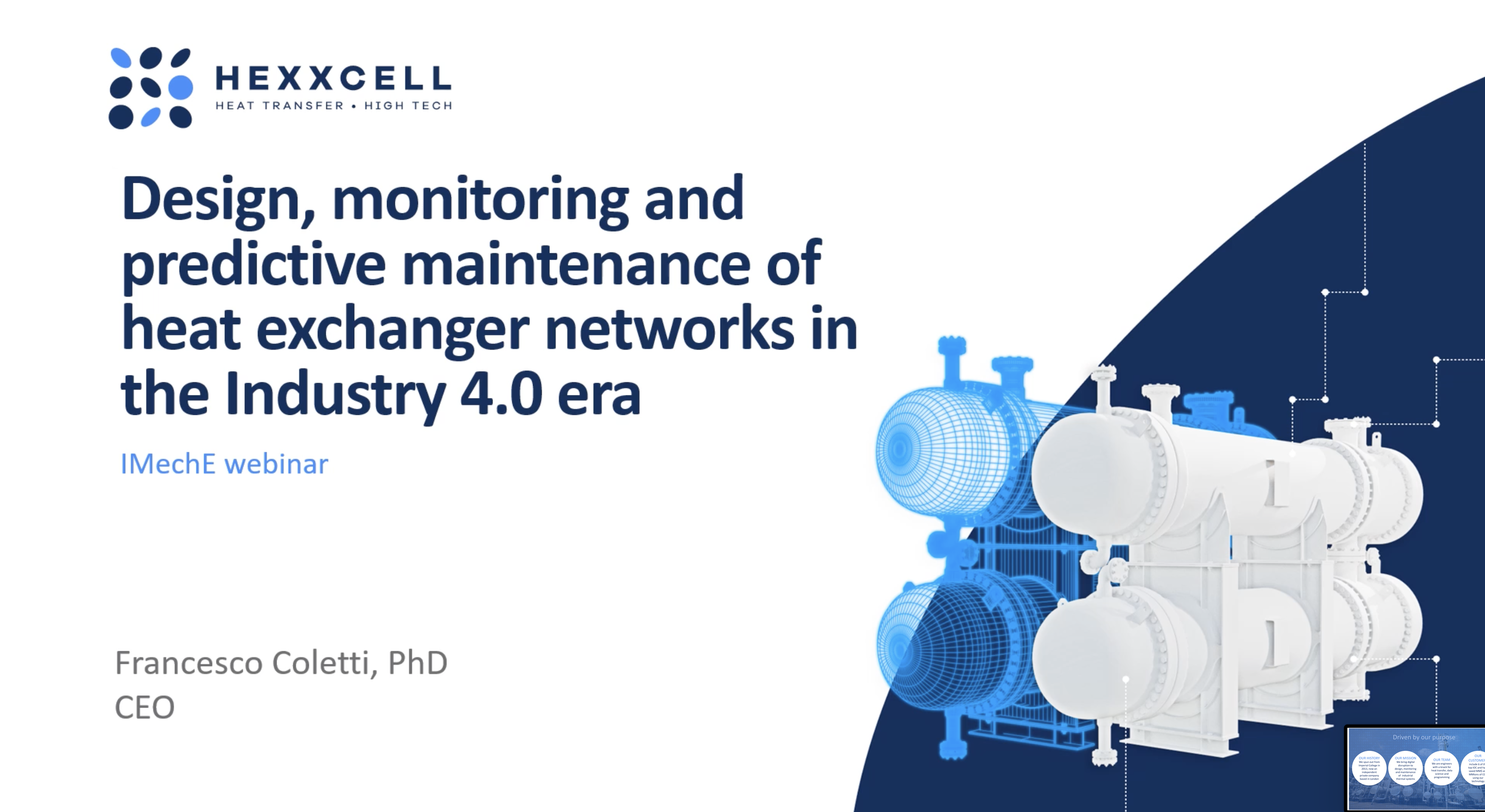
Desing, Monitoring and Predictive Maintenance of heat exchangers networks in the Industry 4.0 Era.
Heat Exchanger Desin Handbook (HEDH) Webinars
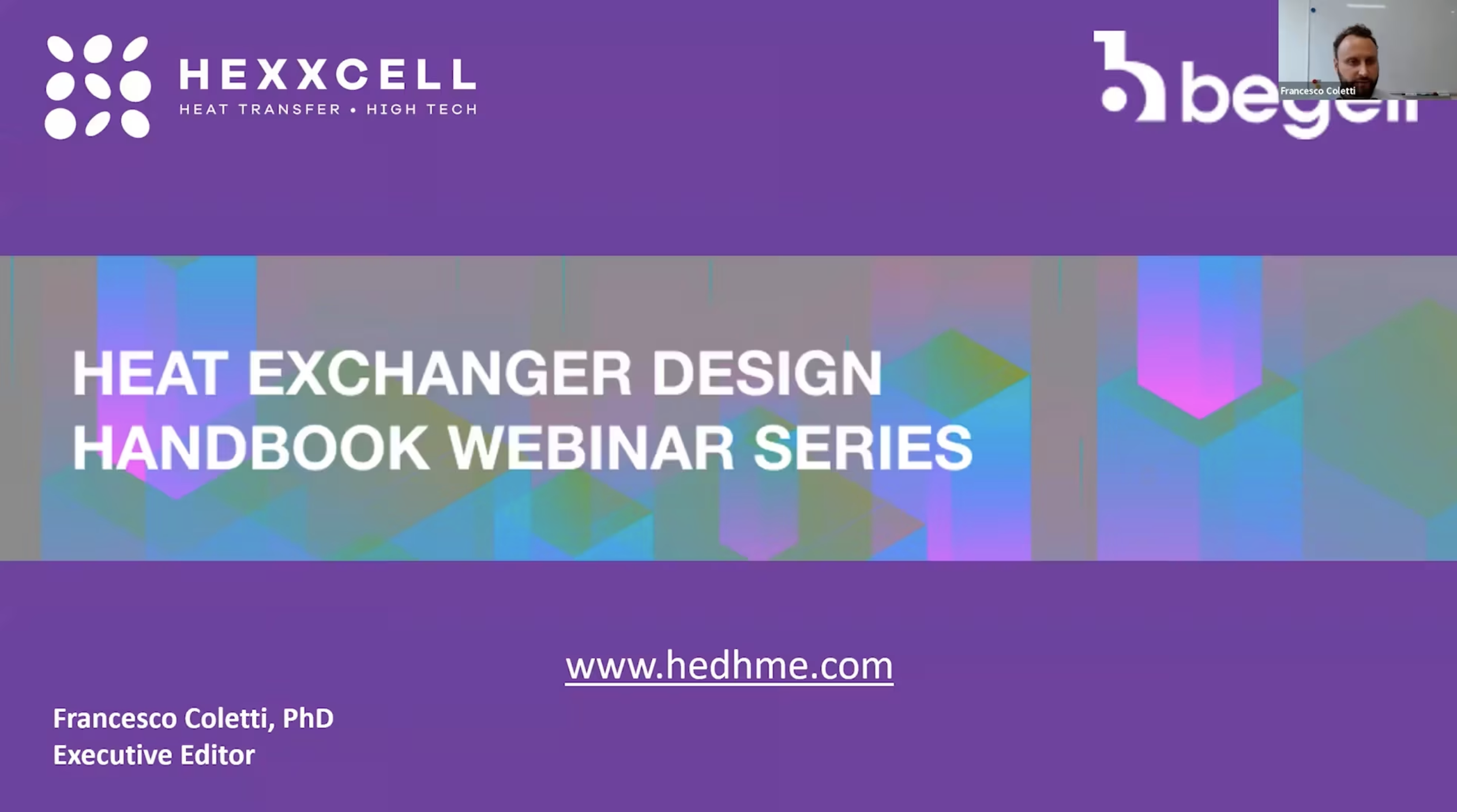
How heat exchangers foul and how to design effectively
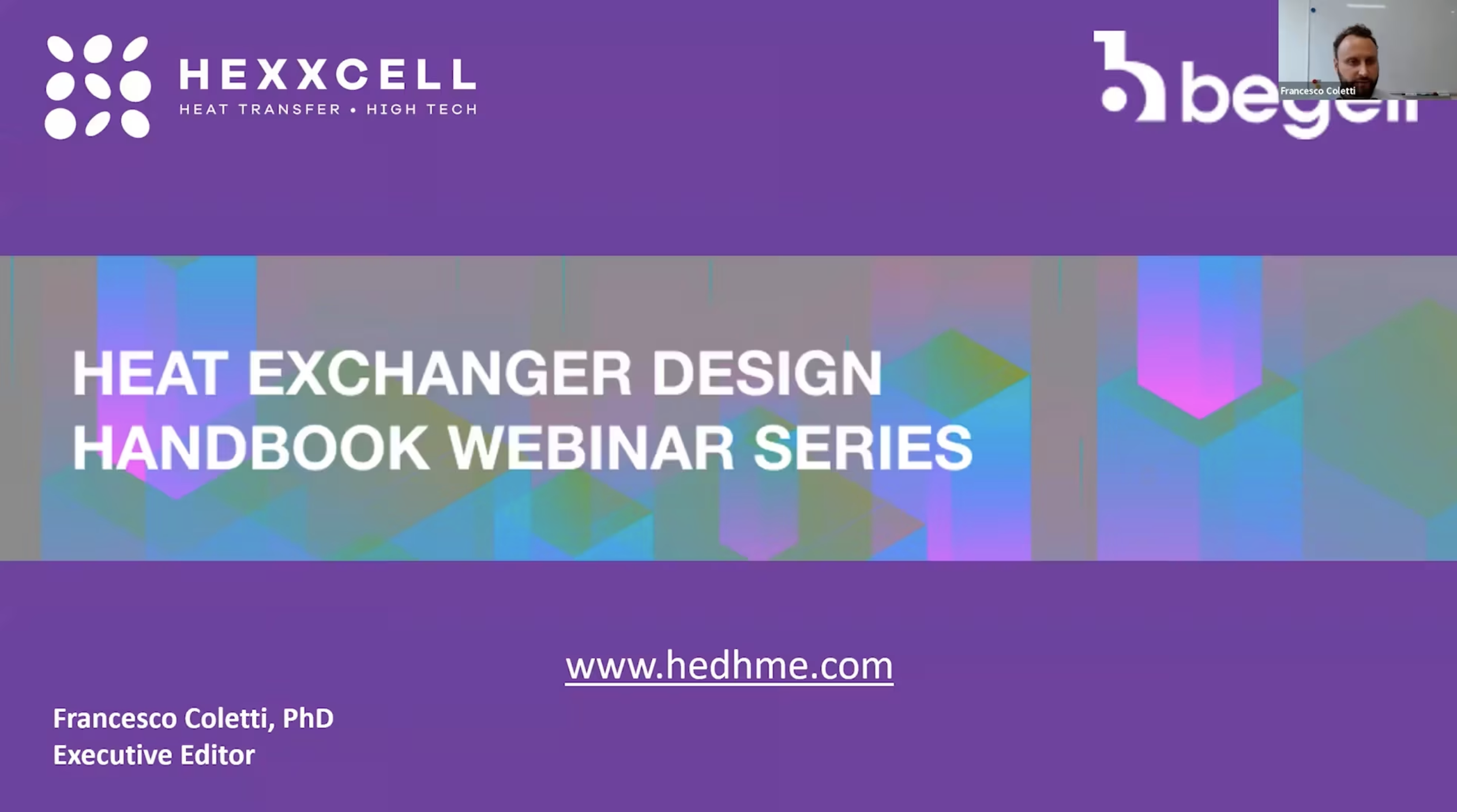
High temperature thermal energy storage
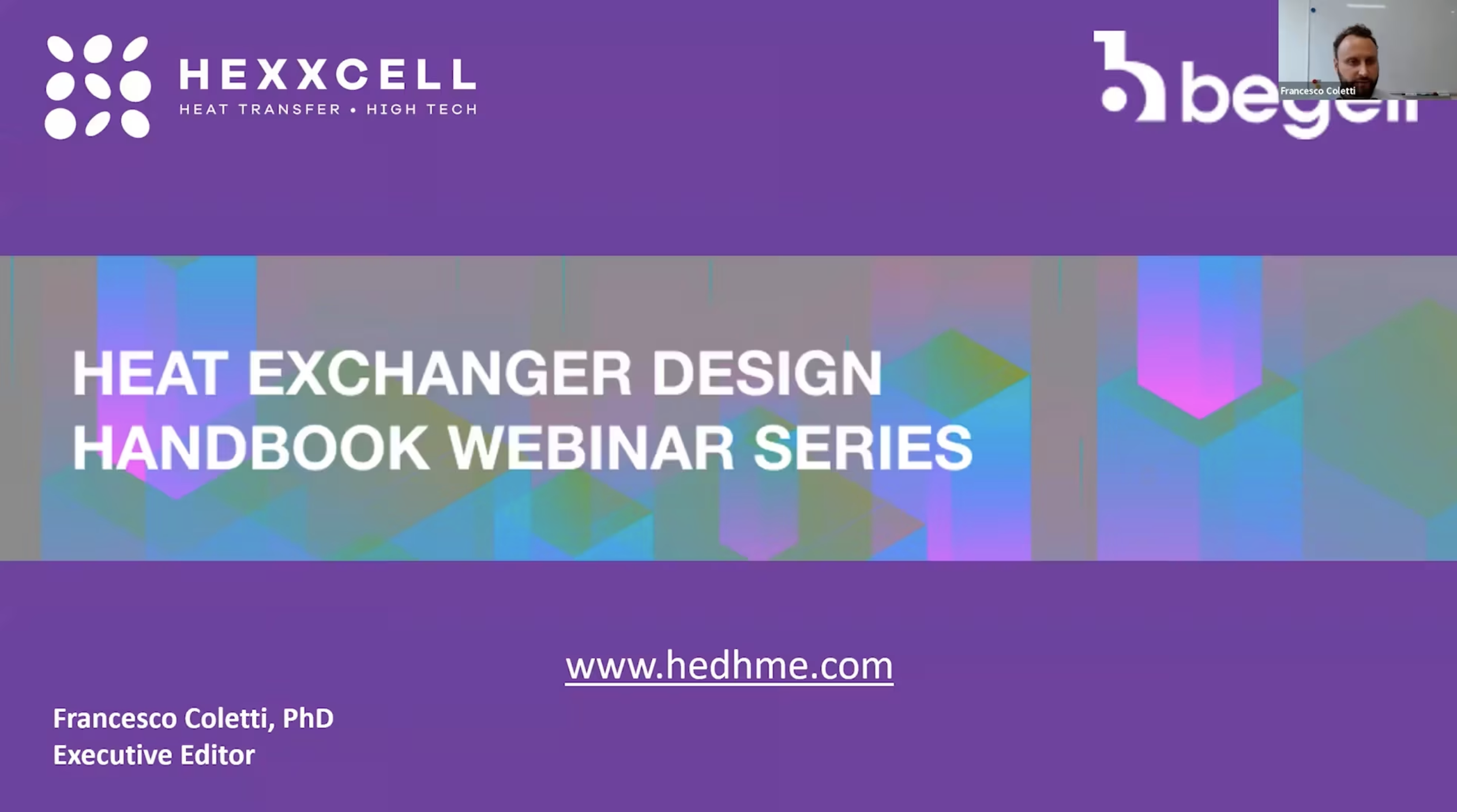
Cleaning of Heat Exchangers
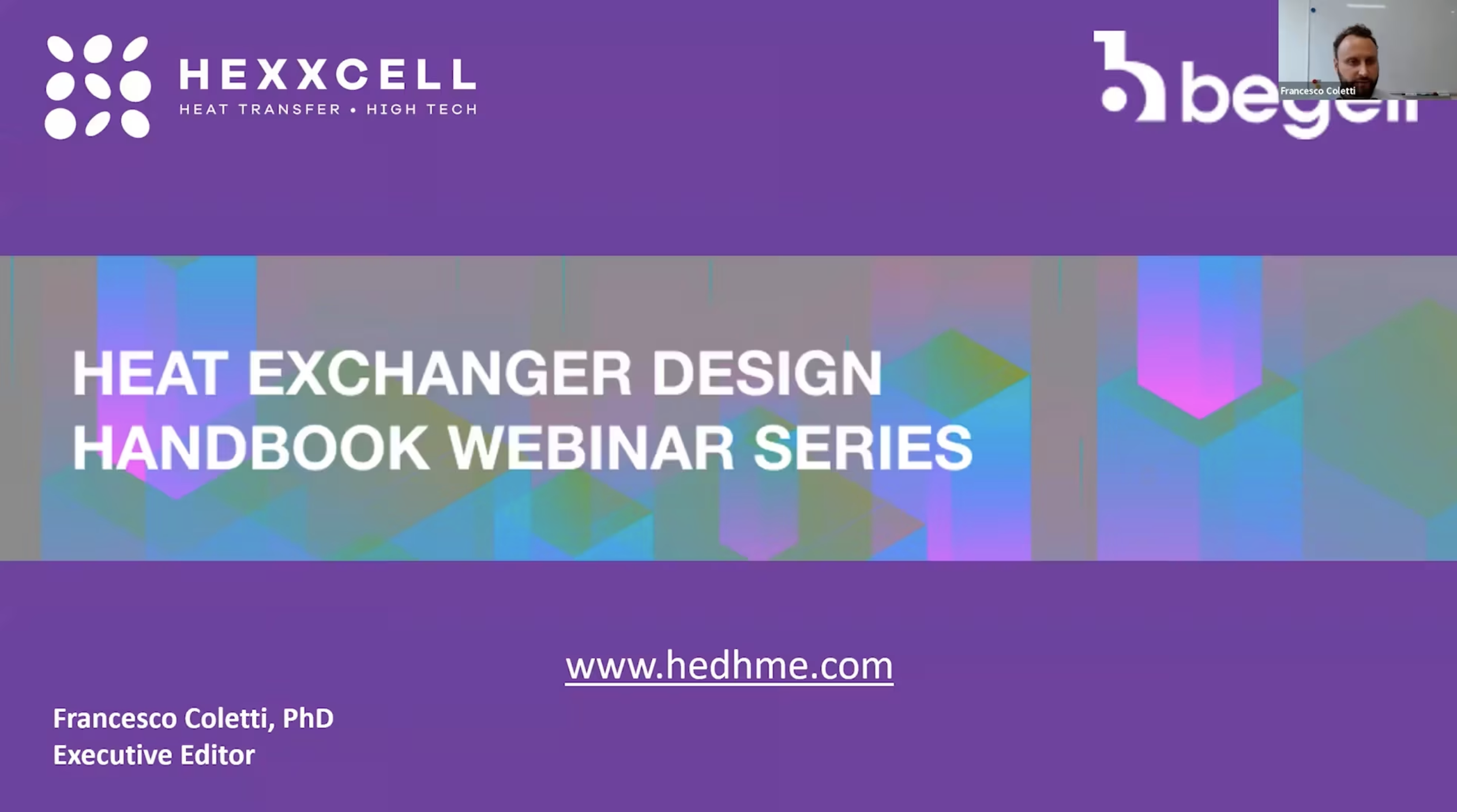
Operational Issues Caused by Incorrect Heat Exchanger Design Choices
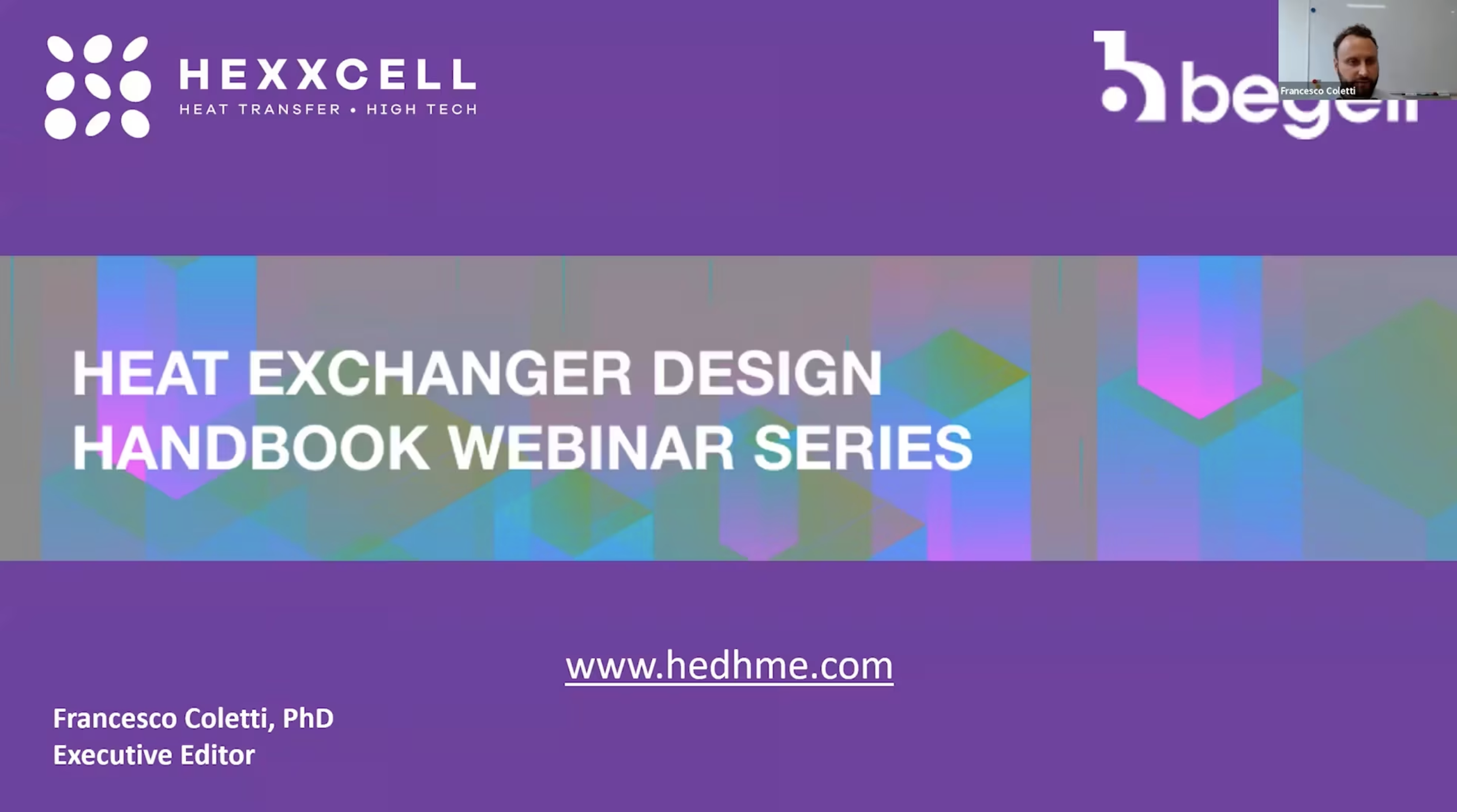
Additive Manufacturing for thermal science: how can we design innovative and efficient heat exchangers?
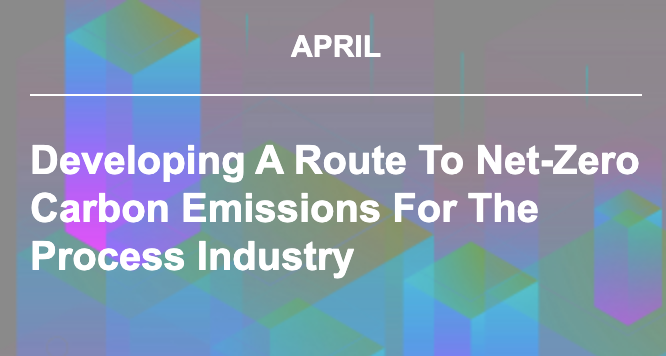
Developing a Route to Net-zero Carbon Emissions for the Process Industry